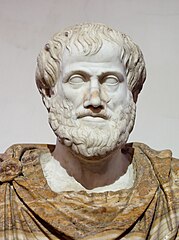
Analysts and marketers do a lot of naming and classifying — This is a That — in order to communicate distinctions among the many available products and technologies. The categorization practice dates back at least to Aristotle, who created taxonomies for scientific classification. Classification helps us describe and predict.
Granted, software category definitions and boundaries are often disputed. Where industry analysts seek to clarify, often a marketer’s aim is to create differentiation, via a unique category label, for a product that is otherwise little different from a competitor’s.
An example: The terms “text analytics” and “text mining” are largely interchangeable. They name the same set of methods, software tools, and applications. Their distinction stems primarily from the background of the person using each — “text mining” seems most used by data miners, and “text analytics” by individuals and organizations in domains where the road to insight was paved by business intelligence tools and methods — so that the difference is largely a matter of dialect.
Sometimes, by contrast, it helps to stress a difference in the import of two terms that the majority use interchangeably. Text Analysis and Text Analytics are two such terms. Both describe creation of insight, via machine processing, from in-the-wild text found in diverse online, social, personal, and enterprise sources and formats. Yet we’d profit by differentiating the two terms, hence this article.
Text Analysis Versus Text Analytics
I’ve been describing term usage: A bit of language analysis. Analysis is an examination of structure, composition, and meaning that provides insight to advance some purpose. Analysis may be heuristic, informal, and/or qualitative.
Contrast with analytics, which is algorithmic rather than heuristic. I define analytics as the systematic application of numerical and statistical methods that derive and deliver quantitative information, whether in the form of indicators, tables, or visualizations. Analytics is formal and repeatable.
Now let’s jump from analysis and analytics in general, to text analysis and text analytics. Qualitative vs. quantitative is perhaps the differentiator we seek, along with a judgment whether the text itself is the object of interest, or whether the text is merely a container for what interests us, namely extractable information content.
Information content (of text): We’re talking entities, facts, relationships, opinion, emotion, intent, identity, and events. Deconstruct a news excerpt, “For fiscal 2013, Oracle reported earnings of $17.6 billion… Oracle president Safra Catz touted operating margins of 47 percent for the fiscal year,” and a hotel-review snippet, “Not a bad choice if gambling is your thing and you don’t mind the ever-present stench of cigarettes… Next time we’re in Vegas, I think we will go for MGM Resorts’ more modern properties,” and you’ll find examples of that good stuff. Your aim is to convert text into data. Crunch a few hundred of these messages (or a few hundred million: this is the big-data era) to compute indicators, spot trends, set alerts, populate a dashboard, and derive predictive and prescriptive models. For good measure, join extracted information with transactional records and demographic profiles and reference data. Abracadabra: You’re doing text analytics.
(Want to learn more? Check out my next Sentiment Analysis Symposium, March 5-6, 2014 in New York, where I’ll have speakers including Stephen Pulman of Oxford Univeristy, on Bleeding Edge Natural Language Processing; Aloke Guha of start-up Cruxly, on Real Time Intent and Sentiment Analysis; Rosalind Picard of the MIT Media Lab on Emotion Recognition; and Sarah Biller, Capital Market Exchange, on Trading Signals from Investor Sentiment.)
In text analysis, the object of interest is the text itself. The analysis describes, and derives qualitative properties from, a document or message (or a collection of documents). Text analysis obtains a text’s salient attributes and characteristics. Text analysis might discern:
- Language: Is a product review in Parisian or Québécois French?
- Genre: Is an e-mail message a product inquiry, service request, complaint, sales order, or something else?
- Descriptive metadata: Authorship, title, publication/posting date.
- Tone: Is Angry, happy, sad, insulting, complimentary, or 50 other shades of mood. (Huffington Post factors in automatically detected tonality in its automated comment moderation, which CTO John Pavley will speak about at the sentiment symposium.)
- Literary style: What level reader is an article written for?
- Author identity or demographic classification: What do use of slang, idiom, topic, and topical references tell you about the sex, age, ethnicity, and geographic origin of the person posting?
- Signals such as intent: What do the wording and syntax of a tweet say about hopes and plans, that individually and in the aggregate, represent opportunities and threats?
Admittedly, the boundary here, between text analysis and text analytics, is not rigid. Forms of analytics may enable functions where, as I put it above, the text itself is the object of interest. Two examples of text processing needs that rely on analysis are machine translation — accurate translation relies on large-scale statistical analysis of language, at the phrase level — and automated abstracting and summarization, where a text is shortened in ways that accurately reflect the sense or narrative of the longer text. Add to these two text transformations two others, compression and encryption, powered by analytical algorithms but about form rather than insights.
So we have text analytics on the one hand — text as data, fueling quantitative methods to communicate business-required insights — and text analysis on the other, techniques that characterize and describe a text itself. If the distinction is meaningful for you: Run with it. If you see me as splitting hairs, well I hope I’ve at least imparted a sense what text analysis/analytics can do for business, whatever your goal.