Interactions, facts, and feelings shape our relationships. A truism: It’s not what you say, but how you say it. Expressions matter, as do the sentiment behind each encounter and the emotions raised. Emotion is entwined with the literal meaning of words used.
This fact/feeling principle applies to both inter-personal and business relationships. “Emotional and factual appeals cannot be easily separated,” writes Nigel Hollis of Kantar Millward Brown in an analysis of advertising approaches. “[A] distinction between emotional and rational is one that exists only in the minds of marketers, not consumers,” according to Hollis.
The fact/feeling equation is central to corporate customer experience (CX) initiatives. CX practitioners map customer journeys that are defined by both the what and the how-did-it-make-you-feel? of customer-brand interactions. “Emotion drives loyalty,” according to CX visionary Bruce Temkin, and loyalty drives profit.
Another truism: You can’t improve what you don’t measure, not systematically, on a corporate scale.
Sentiment Analysis and the Varieties of Emotion AI
Enter sentiment analysis, software technology that quantifies mood, attitude, opinion, and emotion in digital media, in images, video, audio, and text. One subspecies infers emotion via facial-expression analysis. Providers include Affectiva, CrowdEmotion, Eyeris, Kairos, nViso, Noldus Information Technology, RealEyes, and Sightcorp. Another variety analyzes emotion in speech. Check out audEERING, Beyond Verbal, EMOspeech, Good Vibrations, NICE, Verint, and Vokaturi. On the text front, natural language processing (NLP) techniques can identify and extract emotion in online, social, and enterprise sources, delivered by companies that include Clarabridge, Crimson Hexagon, Feedback Ferret, IBM (AlchemyAPI and Watson Tone Analyzer), indico, Receptiviti, and an advisee of mine, Heartbeat AI Technologies.

This article aims to get a handle on the state of emotion analytics — specifically, emotion in text — via an interview with Heartbeat founder Lana Novikova. Lana describes herself as a marketer by training and a market researcher by trade, never satisfied with numbers and observations, always pushing to understand the “deep why” behind consumer (and her own) decisions. She’ll be presenting at LT-Accelerate, a conference I organize, November 21-22 in Brussels, alongside Odile Jagsch, a consultant at global market research consultancy Kantar TNS, topic “The ‘Why’ Behind Customer Loyalty.”
Heartbeat and Emotionally Intelligent Technologies
Seth Grimes> Heartbeat designs “emotionally intelligent technologies.” OK, what’s an “emotionally intelligent technology”?
Lana Novikova> Let’s start with the concept of Emotional Intelligence (EQ), popularized by a psychologist Daniel Goleman in 1990s.
Imagine a newborn human who comes with a basic wiring for recognizing and expressing key emotions, and with an enormous capacity to learn. She can cry or stay calm, smell and turn her head towards her mother’s breast. Once she can see faces, her mirror neutrons start learning and mimicking facial expressions; then she develops more and more capacity to read and express emotions — from touch, to tone & voice recognition, to basic language to more complex if-then scenarios. In a perfect world, she grows into a secure and happy person who can recognize and name a wide range of her own emotions, understands what other people feel from multiple expressions, and has a capacity to express and manage her emotions.
Seth> So you apply the EQ concept to and via technology.
Technology today has a super high IQ — it can beat the best human chess, Jeopardy, and Go players — yet it has a very low EQ. At Heartbeat, we want to be a part of an academic and business community that changes this. Emotionally intelligent technology is never going to feel emotions or express them like our baby can, but it will eventually become very good at perceiving and understanding human emotions from data. One day, this technology might surpass humans in understanding human emotions because it will tap into data that humans can not perceive on their own: biometrics, brain waves, subtle cues from body language and facial expressions, and more.
Seth> Relating the tech to Heartbeat…
We are focusing on training the (metaphorical) technology infant to recognize explicit feelings from language, from text, and to guess the range of emotions it communicates.
Just as some people can intuitively differentiate between many emotions, our growing algorithm can tell what kind of Joy or Anger is expressed in language. There could be as little as 2-3% and as much as 50% affect words and phrases in any given unstructured text. We find these words and assign them to a cluster of emotions. This process mimics how our brain deciphers emotions from language.
Seth> What aspects of emotion does Heartbeat detect and measure? Do you adopt a particular emotion model?
Lana> There are a few models and classifications of emotions developed by brilliant psychologists like Paul Ekman and Robert Plutchik, and even by Human-Machine Interaction Network on Emotion (HUMANE). I was inspired by a more intuitive model of W. G. Parrott (2001), originally described by Shaver in 1987. It has a tree structure and includes Primary, Secondary and Tertiary emotions. I also did a lot of reading about effective neuroscience, and tried to combine Parrott’s model with what I took from the work of J. LeDoux, R. Davidson, and J. Panksepp. Then my “practical life-long quant researcher” side took over and asked, “How is this segmentation going to be useful to a brand of chocolate, or a bank, or a political party?”
We ended with a 2-level clustering of 99 complex emotions and feelings into 9 primary emotions: Joy, Love, Trust, Anger, Fear, Disgust, Sadness, Surprise and Void (which is explicit lack of emotion like in “I don’t care”). We also added Body Sense (positive, negative and neutral) as a way to analyze words and phrases that don’t point to a particular emotion, yet are useful for understanding human perception overall, especially for marketing food and body care products.
Many words and phrases are coded into multiple emotion clusters. Would you agree that there is a large overlap between Disgust and Anger, or Love and Joy, or even Anger and Fear? For example, we put the word “terrific” into both Joy and Fear, and let context decide which emotion it is more likely to represent. This is the most challenging part of our journey — understanding how different context colors “terrific” into happy or unhappy expression. It’s the domain of Machine Learning that needs lots of training data. We are just scratching the service here, but this is also the most exciting part of my job.
Seth> How does the tech work?
Lana> Today, our tech is very simple yet very accurate. It’s called “bag of words”: 8,000+ words and phrases (including negation, metaphor, and other multigrams) professionally coded into categories and validated by skilled psychologists and psycholinguists. Our software consumes unstructured text from survey responses and social media, and produces a set of visualizations and charts in a simple elegant dashboard.
We do our best work when we analyze data from survey responses that focus on people’s feelings. Data like that produces over 30% affect words, and has the context controlled by researcher. Once the report is ready (which is almost instantly), we curate results by removing some words that do not apply to the report. This is how we deal with another industry challenge, ambiguity. Finally, to prove that we are very good at what we do – we show all words and phrases for each Primary emotion. You can click on any word and see exactly how it appeared in the original text – no “black boxes” here. Since our taxonomy reached 5000, our match rate — the percentage of affect words that we recognize — is over 95%. Heartbeat is committed to accuracy, depth, and transparency.

Seth> How is Heartbeat different or better than the competition?
Lana> Heartbeat is different because it was created by a market researcher (myself) who spent hundreds of hours coding open ended survey data. My team built our award winning app for researchers and marketers who appreciate the depth of consumer insight. I love working with good quality data, and would choose quality over quantity any time.
Survey data is under-used and often abused. The art of analyzing good quality text data lies in understanding (a) how to ask a good question, and (b) how to infer meaning from people’s answers. I believe Heartbeat is better for distilling emotions from open-ended survey questions than any other company on the market today including IBM/Alchemy and other powerful APIs. They can do a lot of advanced text analytics with huge amounts of data. We made it simple, transparent, and fun. Just check out our dashboards — clients love it! Another big differentiator is that we are 100% focused on emotions — not sentiment or basic emotions, but fine-grained feelings. Our reports can be useful for anyone – from a CEO and CMO to a brand manager to a CX analyst to an agency creative director.
Seth> How do Heartbeat emotion text analytics findings complement or compare with insights discovered via neuroscience and biometrics, via facial image recognition and brain-activity measurement?
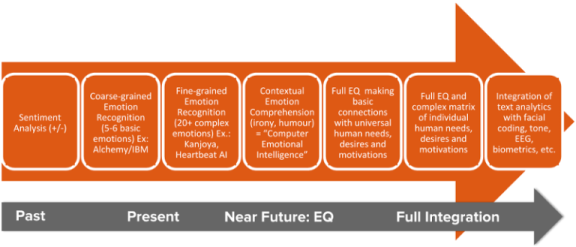
Lana> I strongly believe in cooperation over competition. I think putting our best technologies together will create a better future for our businesses and for this planet. A graphic will communicate how I see the future of emotion AI integration. (See the Heartbeat-provided image.)
Seth> Could you please sketch out 2 or 3 use cases? What’s your target market — clients and applications?
Lana> Let’s start with the customer, the consumer or shopper. What do all customers have in common? They’re human, they feel emotions all the time, and those emotions drive many (if not most) of their decisions.
Why not measure emotions on every step of your customer journey? It’s not easy to put an eye tracking and EEG device on thousands of people, but it’s easy to ask one simple question, “Please share a few words that best describe how you feel about X.” This natural human question fits nicely into any survey and customer feed-back tool, and it’s surprisingly powerful. It not only invites a wide range of un-aided and un-biased feelings on the subject, but it can also aid at predicting what people will do in the future.
We’re a start-up, but we have already published two case studies to show the predictive power of emotions measured with HEARTBEAT emotion analytics: banking and political elections. The best application of HEARTBEAT is in on-going customer experience measurement and foresight.
Seth> What’s on your product roadmap?
Lana> We are driving in the fast lane! We launched our first prototype last December, and won an international startup competition (Insight Innovation Competition by GreenBook) in March 2016 in Amsterdam. All Spring and Summer, we tested our tool with some of the best research companies in the world. Finally, we launched enterprise SaaS this fall, which will enable anyone — brands, market-research suppliers, consultancies, marketing agencies — to access the engine. No more manual coding: leave it to a machine, fast and accurate.
Next, we’re venturing into a long complex journey of NLP and machine learning, to crack the challenge of context and meaning.
Here’s a quote that resonates with me, especially when it comes to trying to solve one of the biggest puzzles in the Universe, the puzzle of human consciousness: “The most influential thinkers in our own era live at the nexus of the cognitive sciences, evolutionary psychology, and information technology.” That’s New York Times columnist David Brooks.
The mission of Heartbeat Ai is to design emotionally intelligent technologies and tools to help machines understand peoples’ feelings and improve our emotional wellbeing. I don’t know exactly how we’ll get there in the end, but I know that we are on the right track today.
Seth> Thanks Lana!
Readers, I’ve written-up a couple of other emotion analytics interviews — an IBM Watson blog contribution, Sentiment, Emotion, Attitude, and Personality, via Natural Language Processing, based on a conversation with IBMer Rama Akkiraju and On Facial Coding, Emotion Analytics, and Emotion Aware Applications with Affectiva principal scientist Daniel McDuff — and of course you can learn more about Lana’s Heartbeat work at LT-Accelerate in November. If you can swing a trip to Brussels, see you there!