“Photos are the atomic unit of social platforms,” asserted Om Malik, writing last December on the “visual Web. “Photos and visuals are the common language of the Internet.”
There’s no disputing visuals’ immediacy and emotional impact. That’s why, when we look at social analytics broadly, and in close-focus at sentiment analysis — at technologies that discern and decipher opinion, emotion, and intent in data big and small — we have to look at the use and sense of photos and visuals.
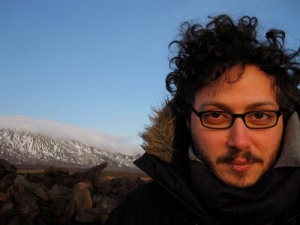
Francesco D’Orazio, chief innovation officer at UK agency FACE, vice president of product at FACE spin-off Pulsar, and co-founder of the Visual Social Media Lab, has been doing just that. Let’s see whether we can get a sense of image understanding — of techniques that uncover visuals’ content, meaning, and emotion — in just a few minutes. Francesco D’Orazio — Fran — is up to the challenge. He’ll be presenting on Analysing Images in Social Media in just a few days (from this writing) at the Sentiment Analysis Symposium, a conference I organize, taking place July 15-16 in New York. And Fran has gamely taken a shot at a set of interview question I posed to him. Here, then, is Francesco D’Orazio’s explanation how —
How to Extract Insight from Images
Seth Grimes> You’ve written, “Images are way more complex cultural artifacts than words. Their semiotic complexity makes them way trickier to study than words and without proper qualitative understanding they can prove very misleading.” How does one gain proper qualitative understanding of an image?
Francesco D’Orazio> Images are fundamental to understand social media. Discussion is interesting, but it’s the window into someone’s life that keeps us coming back for more.
There are a number of frameworks you can use to analyse images qualitatively, sometimes in combination, from iconography to visual culture, visual ethnography, semiotics, and content analysis. At FACE, qualitative image analysis usually happens within a visual ethnography or content analysis framework, depending on whether we’re analysing the behaviours in a specific research community or a phenomenon taking place in social media.
Qualitative methods help you understand the context of an image better than any algorithm does. By context I mean what’s around the image, who’s the author, what is the mode and site of production, who’s the audience of the image, what’s the main narrative and what’s around it, what does the image itself tell me about the author, but also, and fundamentally, who’s sharing this image, when and after what, how is the image circulating, what networks are being created around it how is the meaning of the image mutative as it spreads to new audiences, etc., etc.
Seth> You refer to semiotics. What good is semiotics to an insights professional?
Francesco> Professor Gillian Rose frames the issue nicely by studying an image in 4 contexts: the site of production, the site of the image, the site of audiencing and the site of circulation.
Semiotics is essential to break down the image you’re analysing into codes and systems of codes that carry meaning. And if you think about it, semiotics is the closest thing we have in qualitative methods to the way machine learning works: extracting features from an image and then studying the occurrence and co-occurrence of those features in order to formulate a prediction, or a guess to put it bluntly.
Could you please sketch the interesting technologies and techniques available today, or emerging, for image analysis?
There are many methods and techniques currently used to analyse images and they serve hundreds of use cases. You can generally split these methods between two types: image analysis/processing and machine learning.
Image analysis would focus on breaking down the images into fundamental components (edges, shapes, colors etc.) in order to perform statistical analysis on their occurrence and based on that make a decision on whether each image contains a can of Coke. Machine learning instead would focus on building a model from example images that have been marked as containing a can of Coke. Based on that model, ML would guess, for instance, whether the image contains a can of Coke or not, as an alternative to following static program instructions. Machine learning is pretty much the only effective route when programming explicit algorithms is not feasible because, for example, you don’t know how the can of Coke is going to be photographed. You don’t know what it is going to end up looking like so you can’t pre-determine the set of features necessary to identify it.
What about the case for information extraction?
Having a set of topics attached to an image means you can explore, filter, and mine the visual content more effectively. So for example, if you are an ad agency, you want to set your next ad in a situation that’s relevant to your audience. You quantitatively assess pictures, a bit like a statistical mood-board to this end. We’re working with AlchemyAPI on this and it’s coming to Pulsar in September 2015.
But topic extraction is just one of the visual research use cases we’re working on. We’re planning the release of Pulsar Vision, a suite of 6 different tools for visual analysis within Pulsar ranging from extracting text from an image, identifying the most representative image in a news article, blog post or forum thread, face detection, similarity clustering, and contextual analysis. This last one is one of the most challenging. It involves triangulating the information contained in the image with the information we can extract from the caption and the information we can infer from the profile of the author to offer more context to the content that’s being analysed (brand recognition + situation identification + author demographic), e.g., when do which audiences consume what kind of drink in which situation?
In that Q1 quotation above, you contrast the semiotic complexity of words and of images. But isn’t the answer, analyze both? Analyze all salient available data, preferably jointly or at least with some form of cross-validation?
Whenever you can, absolutely yes. The challenge is how you bring the various kinds of data together to support the analyst to make inferences and come up with new research hypothesis based on the combination of all the streams. At the moment we’re looking at a way of combining author, caption, engagement and image data into a coherent model capable of suggesting for example Personas, so you can segment your audience based on a mix of behavioural and demographics traits.
You started out as a researcher and joined an agency. Now you’re also a product guy. Compare and contrast the roles. What does it take to be good at each, and at the intersection of the three?
I started as a social scientist focussed on online communication and then specialised in immersive media, which is what led me to study the social web. I started doing hands-on research on social media in 1999. Back then we were mostly interested in studying rumours and how they spread online. Then I left academia to found a social innovation startup and got into product design, user experience, and product management. When I decided to join FACE, I saw the opportunity to bring together all the things I had done until then — social science, social media, product design, and information design — and Pulsar was born.
Other than knowing your user really well, and being one yourself, being good at product means constantly cultivating, questioning, and shaping the vision of the industry you’re in, while at the same time being extremely attentive to the details of the execution of your product roadmap. Ideas are cheap and can be easily copied. You make the real difference when you execute them well.
Why did the agency you work for, FACE, find it necessary or desirable to create a proprietary social intelligence tool, namely Pulsar?
There are hundreds of tools that do some sort of social intelligence. At the time of studying the feasibility of Pulsar, I counted around 450 tools including free, premium, and enterprise software. But they all shared the same approach. They were looking at social media data as quantitative data, so they were effectively analysing social media in the same way that Google Analytics analyses website clicks. That approach throws away 80% of the value. Social data is qualitative data on a quantitative scale, not quantitative data, so we need tools to be able to mine the data accordingly.
The other big gap in the market we spotted was research. Most of the tools around were also fairly top line and designed for a very basic PR use case. No one was really catering for the research use case — audience insights, innovation, brand strategy, etc. Coming from a research consultancy, we felt we had a lot to say in that respect so we went for it.
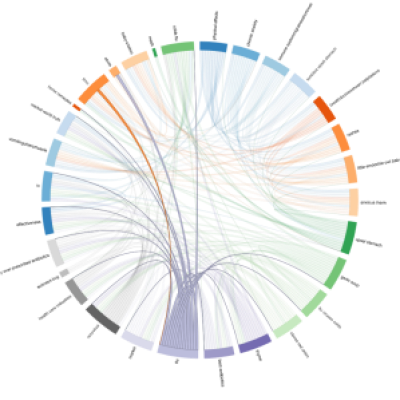
Please tell us about a job that Pulsar did really well, that other tools would have been hard-pressed to handle. Extra points if you can provide a data viz to augment your story.
Pulsar introduced unprecedented granularity and flexibility in exploring the data (e.g. better filters, more data enrichments); a solid research framework on top of the data such as new ways of sampling social data by topic, audience, content, or the ability to perform discourse analysis to spot conversational patterns (attached visual on what remedies British people discuss when talking about flu); a great emphasis on interactive data visualisation to make data mining experience fast, iterative, and intuitive; and generally a user experience designed to make research with big data easier and accessible.
What does Pulsar not do (well), that you’re working to make it do (better)?
We always saw Pulsar as a real-time audience feedback machine, something that you peek into to learn what your audience thinks, does, and looks like. Social data is just the beginning of the journey. The way people use it is changing so we’re working on integrating data sources beyond social media such as Google Analytics, Google Trends, sales data, stock price trends, and others. The pilots we have run clearly show that there’s a lot of value in connecting those datasets.
Human-content analysis is also still not as advanced as I’d like it to be. We integrate Crowdflower and Amazon Mechanical Turk. You can create your own taxonomies, tag content, and manipulate and visualise the data based on your own frameworks, but there’s more we could do around sorting and ranking which are key tasks for anyone doing content analysis.
I wish we had been faster at developing both sides of the platform but if there’s one thing I’ve learned in 10 years of building digital products is that you don’t want to be too early at the party. It just ends up being very expensive (and awkward).
You’ll be speaking at the Sentiment Analysis Symposium on Analysing Images in Social Media. What one other SAS15 talk, not your own, are you looking forward to?
Definitely Emojineering @ Instagram by Thomas Dimson!
Thanks Fran!😸😸